
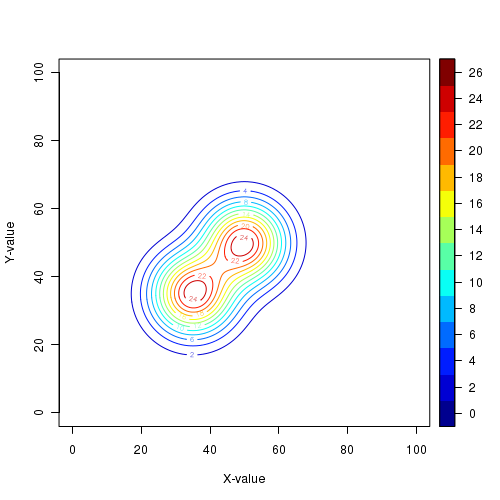

The underlying Bayesian model extends the work of Wadsworth et al. We present MicroBVS, an R package for Dirichlet-tree multinomial models with Bayesian variable selection, for the identification of covariates associated with microbial taxa abundance data. Through simulations, they demonstrate improved performance of their method on selecting covariates when compared to alternative methods, including the penalized likelihood approach of. developed an approach for identifying KEGG orthology pathways that were associated with multivariate count data using a DM regression model with spike-and-slab priors. In this model formulation, unassociated covariates are pushed out of the model and associated covariates’ regression coefficients are freely estimated. A common approach for Bayesian variable selection is to employ a spike-and-slab prior for regression coefficients that depends on a latent inclusion indicator for each covariate. Additionally, they do not accommodate model selection uncertainty while performing selection.Īlternatively, Bayesian variable selection methods are able to accommodate complex, high-dimensional data structures and fully account for model uncertainty over covariate selection. However, the ability of these models to incorporate information about known relations between covariates is limited due to the requirement of complex optimization routines. These models are typically quite efficient and have shown good predictive accuracy. For this, researchers often use penalized likelihood methods to simultaneously estimate regression coefficients and select covariates. Īn important question in human microbiome research is to identify associations between microbial abundance data and clinical covariates, such as KEGG orthology pathways or dietary intake. Microbial abundance data, in particular, have been shown to depend on the evolutionary relations among taxa represented by a phylogenetic tree.
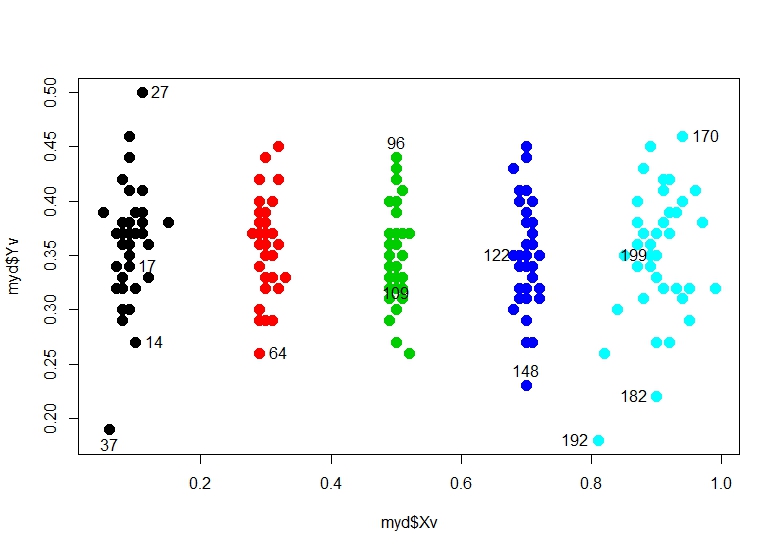
Alternatively, the Dirichlet-tree multinomial model (DTM) inherits the DM’s ability to handle overdispersed data and can model general correlation structures between counts as well as naturally incorporate structural information. However, the DM model only assumes that counts are negatively correlated. Recently, the Dirichlet-multinomial (DM) distribution has been used to model microbial count data, since it can accommodate overdispersion induced by sample heterogeneity and varying proportions among samples. Models developed to investigate microbial taxa abundance data collected on the human microbiome must be able to handle numerous analytical challenges observed in practice, including overdispersion, complex correlation structures, sparsity, high-dimensionality, and known biological information. Characterization of the microbiome is especially critical to the study of chronic diseases such as cancer and diabetes that may be associated with key changes in the microbiome. High-throughput sequencing technologies enable researchers to characterize the composition of the microbiome by quantifying richness, diversity, and abundances (see for a detailed review). The human microbiome is a collection of prokaryotes, archaea, fungi, and viruses which may vary in composition depending on an individual’s health, diet, and environment.
#R help cplot multinom software
While developed to study the human microbiome, our software can be employed in various research applications, where the aim is to generate insights into the relations between a set of covariates and compositional data with or without a known tree-like structure. The underlying Bayesian model accommodates phylogenetic structure in the abundance data and various parameterizations of covariates’ prior probabilities of inclusion. However, available tools frequently ignore evolutionary relations among microbial taxa, potential relations between modulating factors, as well as model selection uncertainty. Numerous analytical tools are available to help identify these relations, oftentimes via automated variable selection methods. Understanding the relation between the human microbiome and modulating factors, such as diet, may help researchers design intervention strategies that promote and maintain healthy microbial communities.
